Notice
OpenModelDB is still in alpha and actively being worked on. Please feel free to share your feedback and report any bugs you find.
The best place to find AI Upscaling models
OpenModelDB is a community driven database of AI Upscaling models. We aim to provide a better way to find and compare models than existing sources.
Found 599 models
RealPLKSR
4x
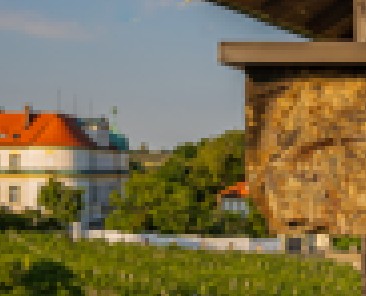
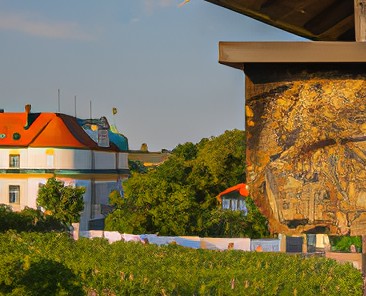
4xNomos2_realplksr_dysample
by Helaman
A 4x model for Pretrained .
4xNomos2_realplksr_dysample
Scale: 4
Architecture: RealPLKSR with Dysample
Architecture Option: realplksr
Github Release
Author: Philip Hofmann
License: CC-BY-0.4
Subject: Photography
Input Type: Images
Release Date: 30.06.2024
Dataset: nomosv2
Dataset Size: 6000
OTF (on the fly augmentations): No
Pretrained Model: 4xmssim_realplksr_dysample_pretrain
Iterations: 185'000
Batch Size: 8
GT Size: 256, 512
Description:
A Dysample RealPLKSR 4x upscaling model that was trained with / handles jpg compression down to 70 on the Nomosv2 dataset, preserves DoF.
Based on the 4xmssim_realplksr_dysample_pretrain I released 3 days ago.
This model affects / saturate colors, which can be counteracted a bit by using wavelet color fix, as used in these examples.
Showcase:
Slowpics
ESRGAN
4x
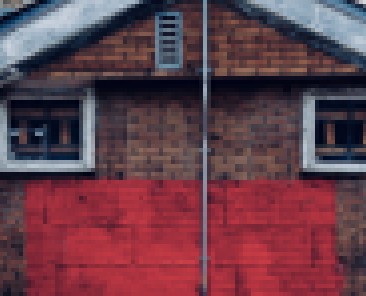
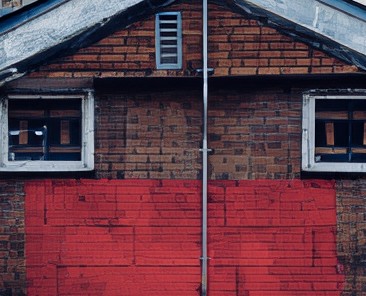
4xNomos2_otf_esrgan
by Helaman
A 4x model for Restoration .
4xNomos2_otf_esrgan
Scale: 4
Architecture: ESRGAN
Architecture Option: esrgan
Github Release Link
Author: Philip Hofmann
License: CC-BY-0.4
Subject: Photography
Input Type: Images
Release Date: 22.06.2024
Dataset: Nomos-v2
Dataset Size: 6000
OTF (on the fly augmentations): Yes
Pretrained Model: RealESRGAN_x4plus
Iterations: 246'000
Batch Size: 8
GT Size: 256
Description:
4x ESRGAN model for photography, trained using the Real-ESRGAN otf degradation pipeline.
Showcase:
Slow Pics 8 Examples
ESRGAN
4x

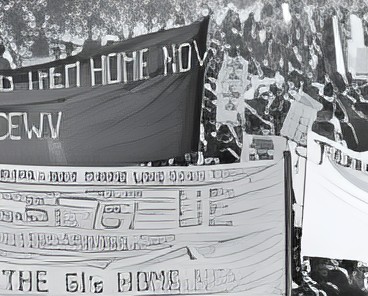
4xNomosWebPhoto_esrgan
by Helaman
A 4x model for Restoration .
I simply wanted to release an ESRGAN model just because I had not trained one for quite a while and just wanted to revisit this older arch for the current series.
4xNomosWebPhoto_esrgan
Scale: 4
Architecture: ESRGAN
Architecture Option: esrgan
Github Release Link
Author: Philip Hofmann
License: CC-BY-0.4
Subject: Photography
Input Type: Images
Release Date: 16.06.2024
Dataset: Nomos-v2
Dataset Size: 6000
OTF (on the fly augmentations): No
Pretrained Model: RealESRGAN_x4plus
Iterations: 210'000
Batch Size: 12
GT Size: 256
Description:
4x ESRGAN model for photography, trained with realistic noise, lens blur, jpg and webp re-compression.
ESRGAN version of 4xNomosWebPhoto_RealPLKSR, trained on the same dataset and in a similiar way.
For more information look into the 4xNomosWebPhoto_RealPLKSR release, and the pdf file in its attachments.
SPAN
2x
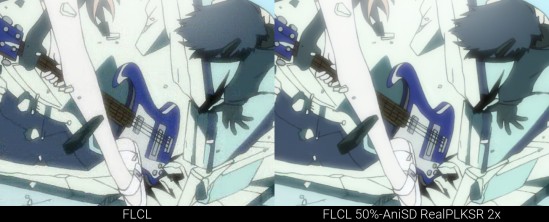
AniSD Suite (Multiple Models)
by Sirosky
## AniSD Suite (15 models)
**Scale:** 2x (1x for AniSD DB)
**Architecture:** SPAN / Compact / SwinIR Small / CRAFT / DAT2 / RPLKSR
**Dataset:** Anime frames. Credits to @.kuronoe. and @pwnsweet (EVA dataset) for their contributions to the dataset!
**Dataset Size:** ~7,000 - ~13,000
AniSD is a suite of 15 (as of time of writing) specialized SISR models trained to restore and upscale standard definition digital anime from ~2000s and onwards, including both both WEB and DVD releases. Faithfulness to the source and natural-looking output are the guiding principles behind the training of the AniSD models. This means avoiding oversharpened output (which can look especially absurd on standard definition sources), minimizing upscaling artifacts, retaining the natural detail of the source and of course, fixing the standard range of issues found in many DVD/WEB release (chroma issues, compression, haloing/ringing, blur, dotcrawl, banding etc.). Refer to the infographic above for a quick breakdown of the available models, and refer to the Github release for further information.
ATD
4x
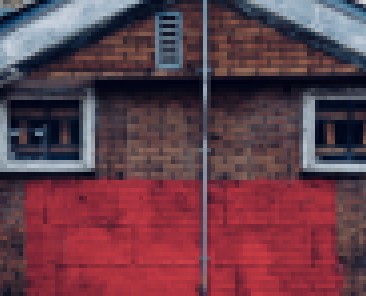
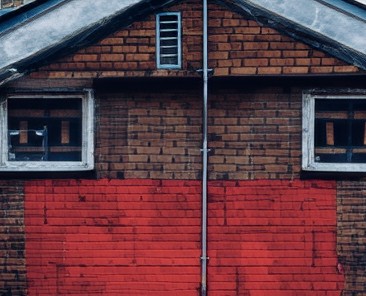
4xNomosWebPhoto_atd
by Helaman
A 4x model for Restoration .
4xNomosWebPhoto_atd
Scale: 4
Architecture: ATD
Architecture Option: atd
Github Release Link
Author: Philip Hofmann
License: CC-BY-0.4
Subject: Photography
Input Type: Images
Release Date: 07.06.2024
Dataset: Nomos-v2
Dataset Size: 6000
OTF (on the fly augmentations): No
Pretrained Model: 003_ATD_SRx4_finetune.pth
Iterations: 460'000
Batch Size: 6, 2
GT Size: 128, 192
Description:
4x ATD model for photography, trained with realistic noise, lens blur, jpg and webp re-compression.
ATD version of 4xNomosWebPhoto_RealPLKSR, trained on the same dataset and in the same way. For more information look into the 4xNomosWebPhoto_RealPLKSR release, and the pdf file in its attachments.
Showcase:
Slow Pics 18 Examples
ESRGAN
2x
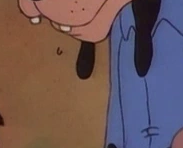
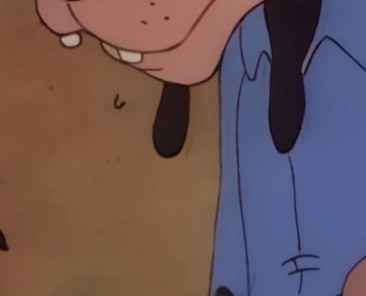
2x Pooh V4
by SharekhaN
A 2x model for Compression Removal, Noise Reduction, Line Correction, MPEG2 / LD Artifact Removal.
This is my first model release. The model was inspired from a personal project which I have been pursuing for some time now, which this model aims to solve. This model will upscale low resolution hand drawn animation from 1970s to 2000. The colors are retained with effective noise control. Details and Textures are maintained to a good degree considering animations. Color Spills are also corrected depending on the colors. Shades of white and yellows have been difficult. It also makes the lines slightly sharper and thinner. This could be a plus depending on your source. The model is also temporally stable across my tests with little observable issues.
**Showcase:**
Images - https://imgsli.com/MjYwNzY1/12/13
Video Sample - https://t.ly/Jp7-w vs Upscale - https://t.ly/PdsKs
Compact
1x
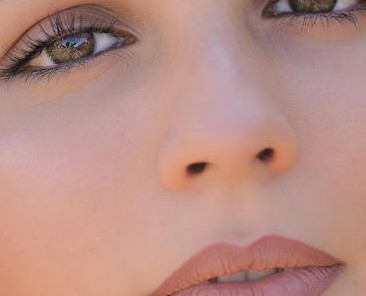
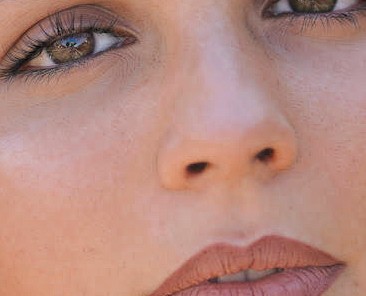
1xSkinContrast-High-SuperUltraCompact
by Brigadeiro
A 1x model designed for skin contrast (although some backgrounds suffer a little modification), also try the other SkinContrast models
Compact
1x
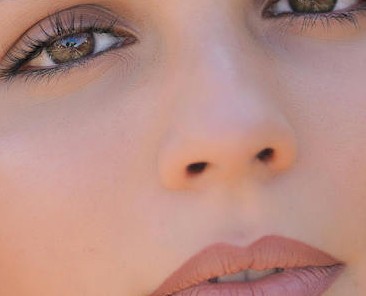
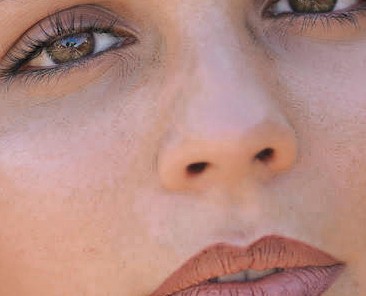
1xSkinContrast-HighAlternative-SuperUltraCompact
by Brigadeiro
A 1x model designed for skin contrast (although some backgrounds suffer a little modification), some images may suffer from the creation of artifacts, High Alternative is the model that generates the most artifacts, also try the other SkinContrast models
Compact
1x
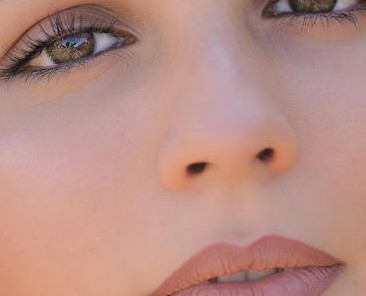
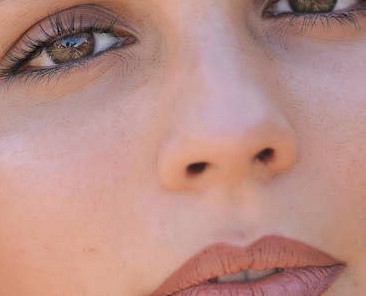
1xSkinContrast-SuperUltraCompact
by Brigadeiro
A 1x model designed for skin contrast (although some backgrounds suffer a little modification), also try the other SkinContrast models
RealPLKSR
4x

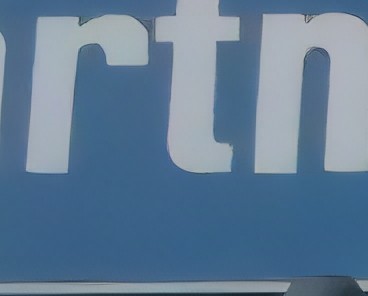
4xNomosWebPhoto_RealPLKSR
by Helaman
A 4x model for Restoration .
4xNomosWebPhoto_RealPLKSR
Scale: 4
Architecture: RealPLKSR
Architecture Option: realplksr
Link to Github Release
Author: Philip Hofmann
License: CC-BY-0.4
Subject: Photography
Input Type: Images
Release Date: 28.05.2024
Dataset: Nomos-v2
Dataset Size: 6000
OTF (on the fly augmentations): No
Pretrained Model: 4x_realplksr_gan_pretrain
Iterations: 404'000, 445'000
Batch Size: 12, 4
GT Size: 128, 256, 512
Description:
short: 4x RealPLKSR model for photography, trained with realistic noise, lens blur, jpg and webp re-compression.
full: My newest version of my RealWebPhoto series, this time I used the newly released Nomos-v2 dataset by musl.
I then made 12 different low resolution degraded folders, using kim's datasetdestroyer for scaling and compression, my ludvae200 model for realistic noise, and umzi's wtp_dataset_destroyer with its floating point lens blur implementation for better control.
I then mixed them together in a single lr folder and trained for 460'000 iters, checked the results, and upon kims suggestion of using interpolation, I tested and am releasing this interpolation between the checkpoints 404'000 and 445'000.
This model has been trained on neosr using mixup, cutmix, resizemix, cutblur, nadam, unet, multisteplr, mssim, perceptual, gan, dists, ldl, ff, color and lumaloss, and interpolated using the current chaiNNer nightly version.
This model took multiple retrainings and reworks of the dataset, until I am now satisfied enough with the quality to release this version.
For more details on the whole process see the pdf file in the attachement.
I am also attaching the 404'000, 445'000 and 460'000 checkpoints for completeness.
PS in general degradation strengths have been reduced/adjusted in comparison to my previous RealWebPhoto models
Showcase:
Slow Pics 10 Examples
Compact
2x
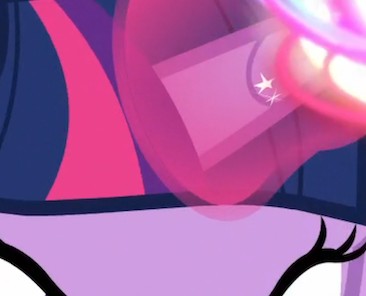
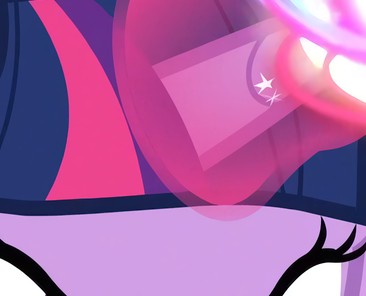
MLP StarSample V1.0
by .derpy.
This is a model for the restoration of My Little Pony: Friendship is Magic, however it also works decently well on all similar art.
It was trained in 2x on ground truth 3840x2160 HRs and 1920x1080 LRs of varying compression, so it is able to upscale from 1080p to 2160p, where its detail retention is great, however it may create noticeable artifacting if looked at closely, like areas of randomly coloured pixels along edges. In 1x or 1.5x (2x upscaled and then downscaled back down) it performs extremely well, almost perfectly in fact, in correcting colours, removing compression, and crisping up lines - and this is the way the model is intended to be used (hence the acronym of its name being "SS", or "supersampling").
**Github Release**
**Showcase:** https://slow.pics/s/1ixqCSjy
SwinIR
4x
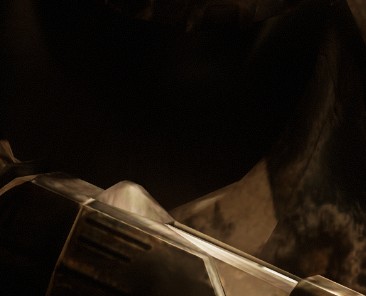
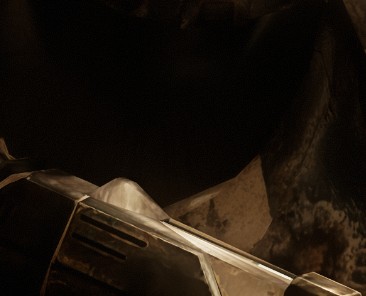
4x-PBRify_UpscalerSIR-M_V2
by Kim2091
This is part of my PBRify_Remix project. This is a much more capable model based on SwinIR Medium, which should strike a balance between capacity for learning + inference speed. It appears to have done so :)